From voxel to diagnosis: AI applications in radiology, part 1
Although extremely valuable and necessary, traditional medical image analysis methods have some limitations. An insufficient number of radiology specialists worldwide means that patients often complain about how long it takes to describe the examination. And as you know, the sooner they get a diagnosis, the better for their further treatment process. AI applications in radiology can significantly enhance radiologists’ efficiency and diagnostic accuracy, serving as a valuable tool to support their daily work.
New technologies, the dynamic development of AI and computer vision, and the increase in computing capabilities have contributed to significant progress in digital medical diagnostics. AI’s ability to recognize patterns that often escape the human eye opens up a new world of diagnostic possibilities. This topic is extremely close to us, so we decided to describe it in a wider aspect. Let’s find out how AI is improving medical image analysis.
Concrete application example: From classification to segmentation
AI applications in radiology are used in a wide range of tasks related to the analysis of medical images. One of the most important areas is classification, i.e., automatic assignment of a specific diagnostic category to images. AI algorithms can classify images according to needs, e.g., determine the type of cancer or the stage of the disease. Automating such routine tasks saves the time of specialists. They can focus on more complex cases that require a bespoke approach.
Another important is image segmentation, i.e., automatic extraction of specific anatomical structures. Analysis of vast amounts of data allows algorithms to detect even subtle changes that may be difficult for the human eye to see. This, in turn, speeds up the diagnostic process and increases its accuracy.
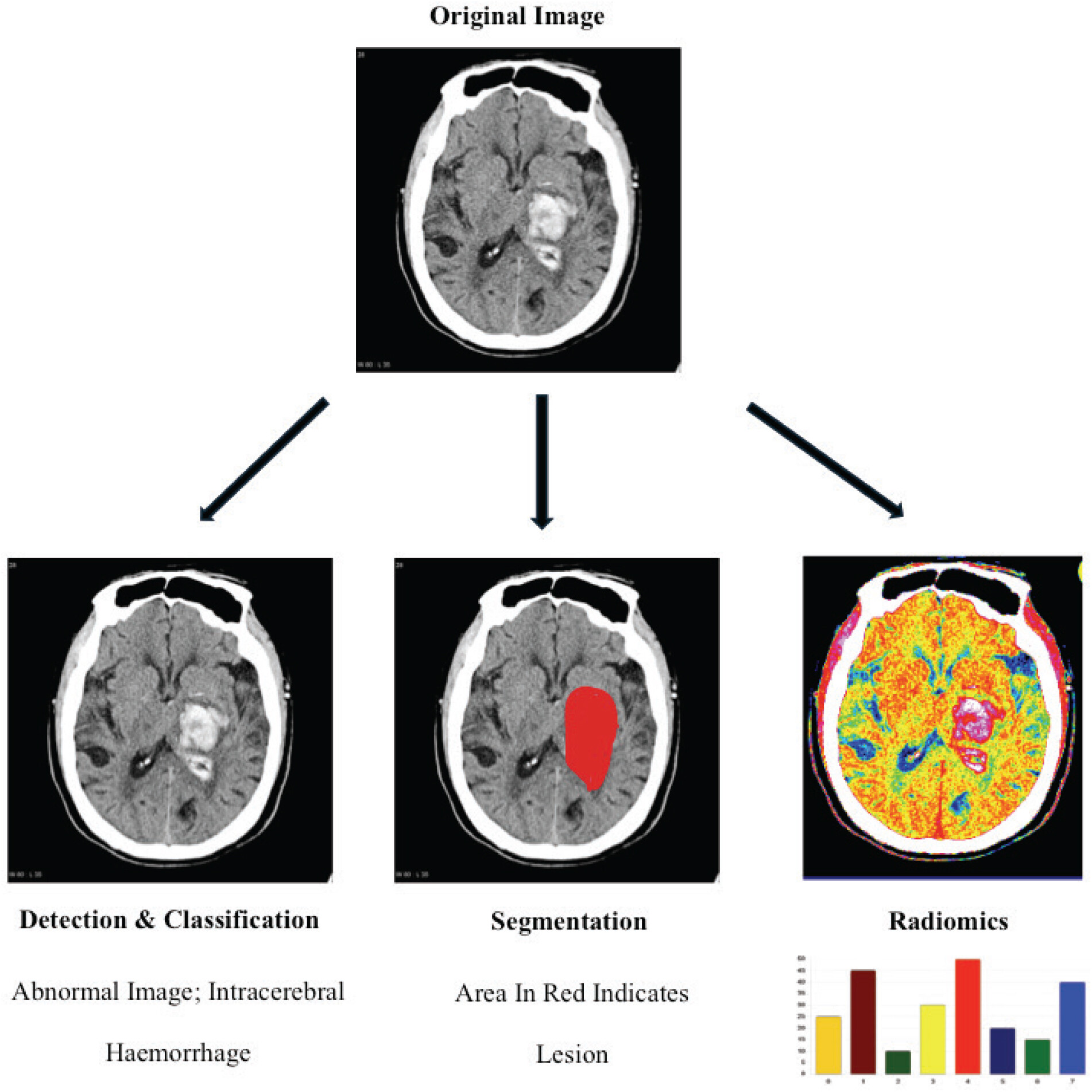
Overview of key clinical use cases for artificial intelligence in medical imaging.
Source: https://www.magonlinelibrary.com/doi/full/10.12968/hmed.2024.0312
Second concrete application example: CT medical imaging
At Graylight Imaging, we’ve developed a new way to create accurate training data for AI models that can segment the aortic valve in non-contrast CT scans. By using image registration, we can semi-automatically generate ground truth data, which allows us to train neural networks to accurately identify the aortic valve in these scans. This makes it easier for doctors to use these models to diagnose heart conditions. We’ve also introduced a new method to measure the accuracy of these segmentation models, which involves comparing the segmented masks from contrast and non-contrast images.
More details about this project can be found in our post: Aortic valve reconstruction in non-contrast computed tomography.
Third concrete application example: Radiomic features
Artificial intelligence algorithms provide an objective, consistent way to assess changes in tumor size or changes in metabolic activity, automating measurements that have traditionally been time-consuming and prone to variability between observers, such as those under the Response Assessment Criteria in Solid Tumors (RECIST).
AI achieves this by using radiomic features multidimensional data extracted from radiological images to build mathematical models adept at detecting subtle changes that indicate a response to treatment. AI algorithms automate the tedious process of manually measuring changes in tumor size and metabolic activity. This allows radiologists to focus on interpreting the results and making clinical decisions.
AI applications in radiology: Challenges and Future
While AI offers many benefits, its use in medicine also comes with some challenges. One of the most important is the need to ensure high quality training data, which is necessary for effective training of algorithms. In addition, there is a need to develop standards for the evaluation and verification of AI-based systems to ensure their safety and effectiveness. Another challenge is the issue of interpretability of AI models. It’s often difficult to understand how an algorithm comes to specific conclusions, making it difficult to trust its results.
Implementing artificial intelligence in clinical practice requires a comprehensive approach, covering both technical and organizational aspects. Investments in appropriate infrastructure, ensuring access to high-quality data and developing appropriate legal regulations are needed. To fully exploit the potential of AI, it is essential that specialists from different fields, from computer scientists to radiologists, work closely together.
The common goal should be to create artificial intelligence systems that will not only improve diagnostics, but also ensure patient safety and comply with the principles of medical ethics. Continuous development of these systems to respond to changing clinical needs is also a priority.
Summary
In conclusion, the role of AI in radiology is transformative, offering advanced diagnostic capabilities. However, this progress prompts consideration of critical issues such as the need for continuous training for healthcare professionals to effectively integrate AI tools and the continuous evaluation of AI systems to ensure that they complement and not replace human expertise. Future advances should aim to harmonise AI technology with clinical practice, ensuring that it remains a supporting tool.
We are on the verge of the fourth industrial revolution, the basis of which is the development of artificial intelligence. The future of medical diagnostics is extremely promising, and artificial intelligence plays and will play a key role in it. This is just the beginning of our deep dive into AI in radiology. Stay tuned for more insights
References:
Najjar, R. Redefining Radiology: A Review of Artificial Intelligence Integration in Medical Imaging. Diagnostics 2023, 13, 2760. https://doi.org/10.3390/diagnostics13172760