Measurement in medical imaging
Unlock the full potential of automated measurement in medical imaging and transform your data into usable, quantitive insights.
We’ve created and continue to develop algorithms for extracting valuable data from medical images in various modalities. With our extensive know-how and technical capabilities, we offer the development of customized solutions tailored to meet your specific needs.
We can create a bespoke system that will provide you with precise and quantitative measurements that are essential for accurate diagnosis, treatment planning, and also disease progression monitoring.
Quantitive data extraction: measurement in medical imaging
The bespoke models we’ve been developing, within measurement in medical imaging for our clients are designed to meet particular needs and requirements. They can measure and analyze as well as provide valuable quantitative data. This technology can be used to detect any lesion, in any anatomical structure or tissue of a single patient.
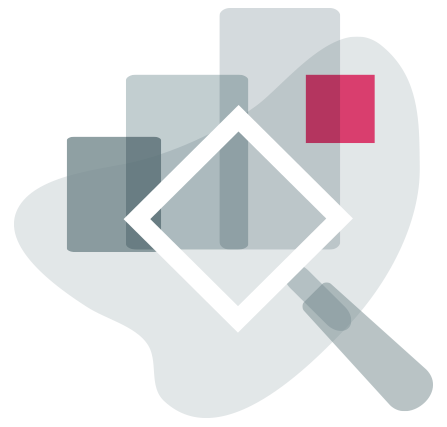
Lesions on scans detection
Accurate detection is essential in medical imaging for precise diagnosis and treatment planning. The advanced algorithms we create can identify single lesions as well as multiple distinct parts of larger, complex lesions. This capability is particularly crucial in cases where a lesion may be multifocal or spread across various regions of an organ.
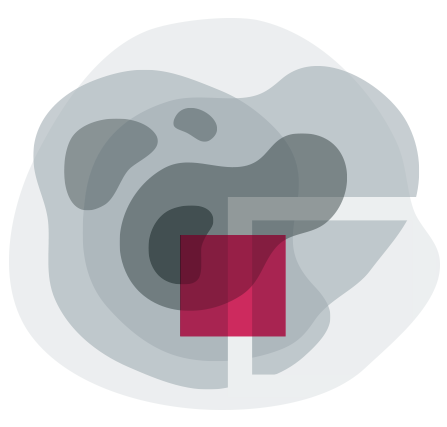
Measure lesions by set criteria
The evaluation of measurable lesions, according to predefined requirements, aims to ensure consistency and accuracy. Advanced solutions can measure specific features such as volume, size, shape, and others. This approach facilitates effective monitoring and diagnosis. Besides it can be a useful tool for monitoring patients in clinical studies.
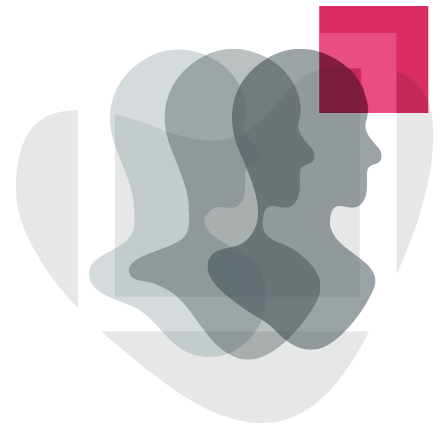
Timed lesion detection
Detecting lesions over time involves identifying new lesions in follow-up scans. In effect, helps track disease progression. By comparing studies over time, algorithms can automatically measure specific areas to monitor treatment response. This is essential not only for the mentioned assessment but also for improving patient care in clinical settings.
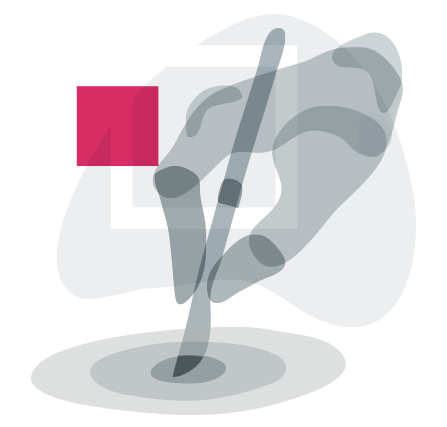
Beyond standard criteria
Evaluation of a patient’s response to treatment can go beyond standard criteria. Medical image analysis algorithms can assessing changes in various areas, providing a more comprehensive understanding of treatment effectiveness. Moreover, this is an opportunity for quantitatively enhanced interpretation.
Proven experience and selected work
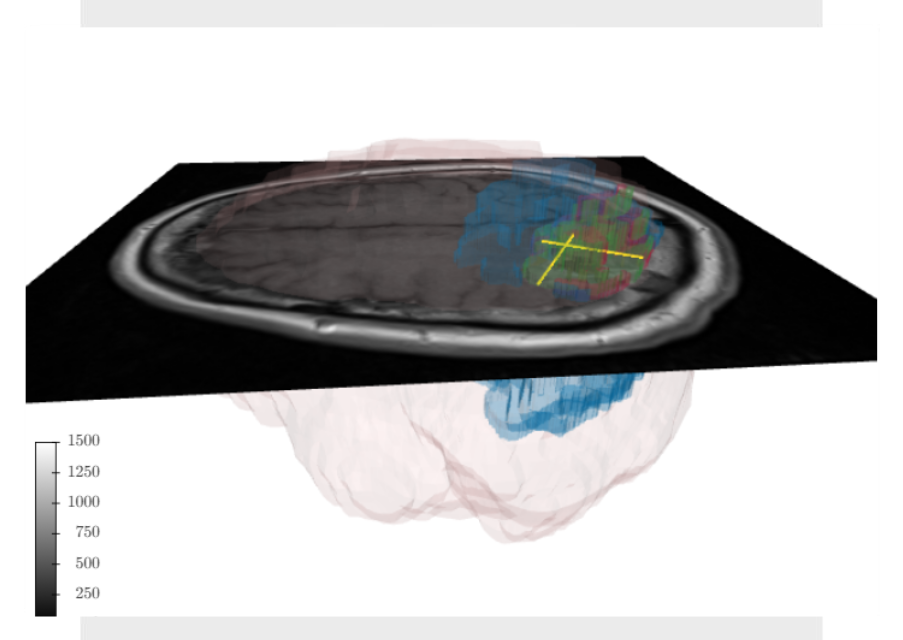
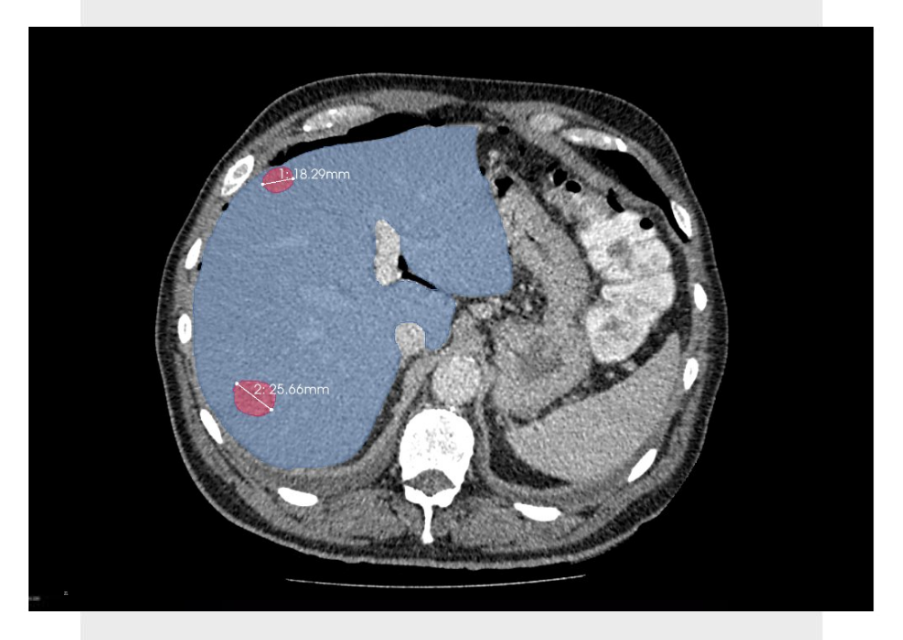
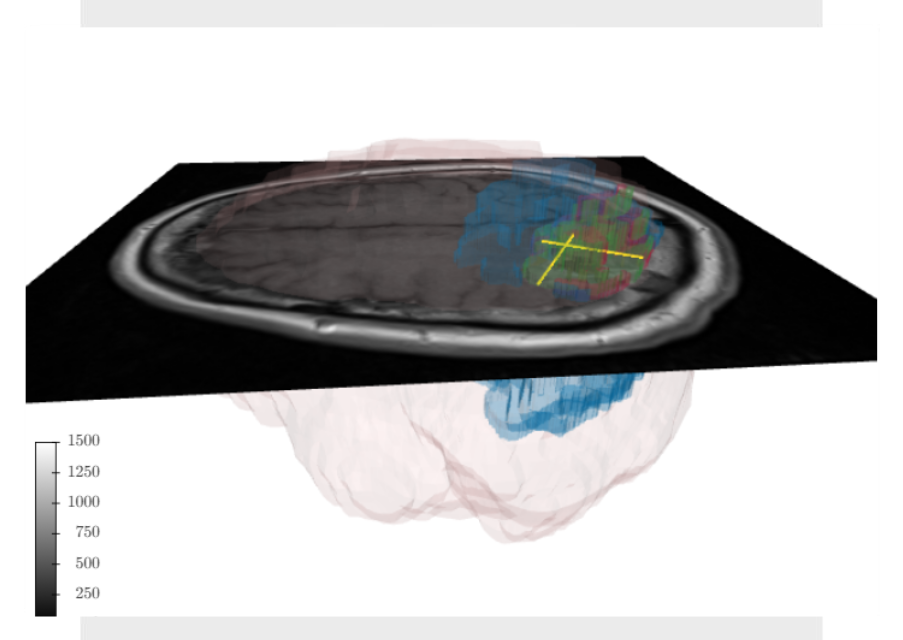
Automated RANO and RECIST+
Fully automated models for precise and comprehensive patient response measurements using RANO and also RECIST criteria to real-world data.
More accurate bidimensional measurements than those typically reported by the majority of human readers – indicating a higher level of precision or detection.
Measurement in medical imaging technology that is capable of much more than the guidelines suggest.
Integration with the segmentation algorithm, in order to all takes place in one step.
Volumetric measurements of predefined regions or subregions and repeatability as well as objectivity of results.
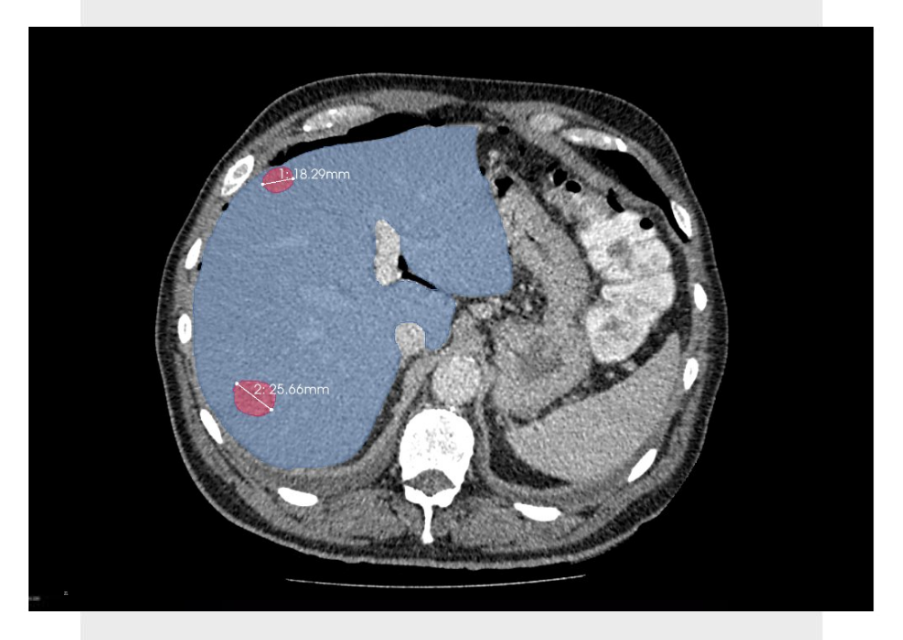
Segmentation with machine learning
Automating the segmentation process using ML solutions – the models we develop contour organs and detect structures localised within organs on various types of examinations.
Accurate, efficient segmentation providing repeatable results and enabling extraction of measurable features of anatomical structures and lesions.
Accuracy of segmentation more than 90% (Sørensen-Dice coefficient of roughly 0.9).
The effective segmentation method proven in prestigious global AI challenges (BraTS and FeTS).
We can provide not only deep learning segmentation model, but also GUI or software if needed.
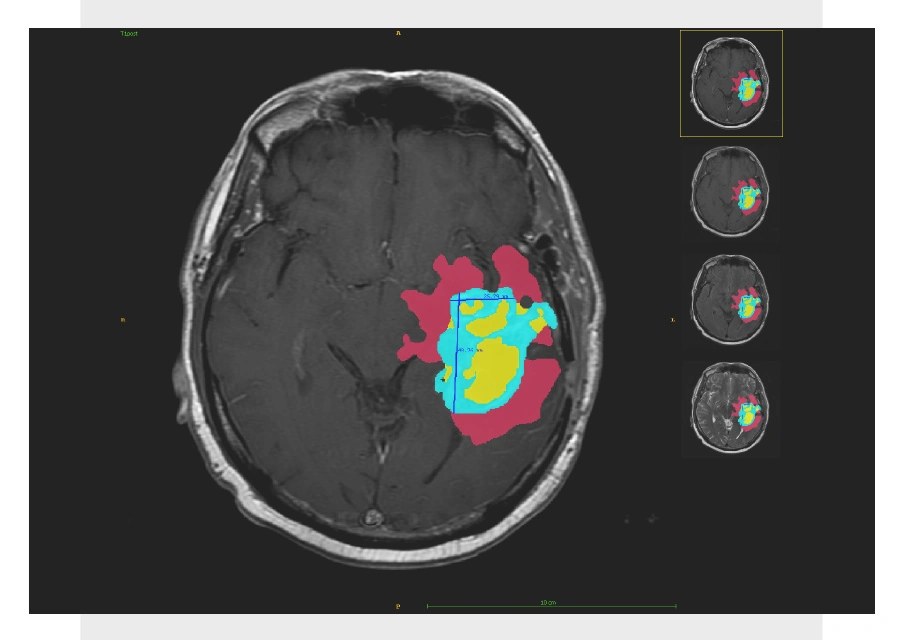
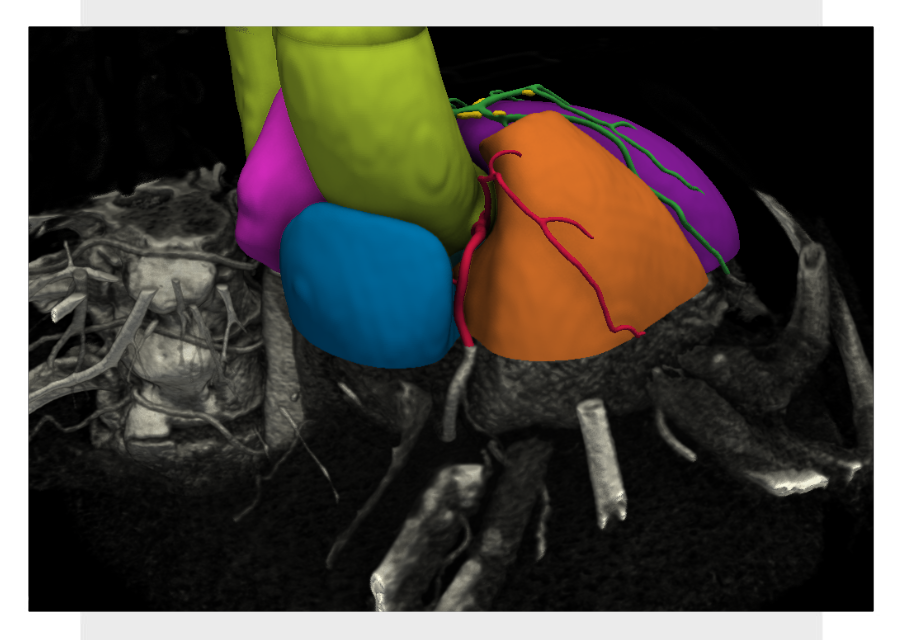
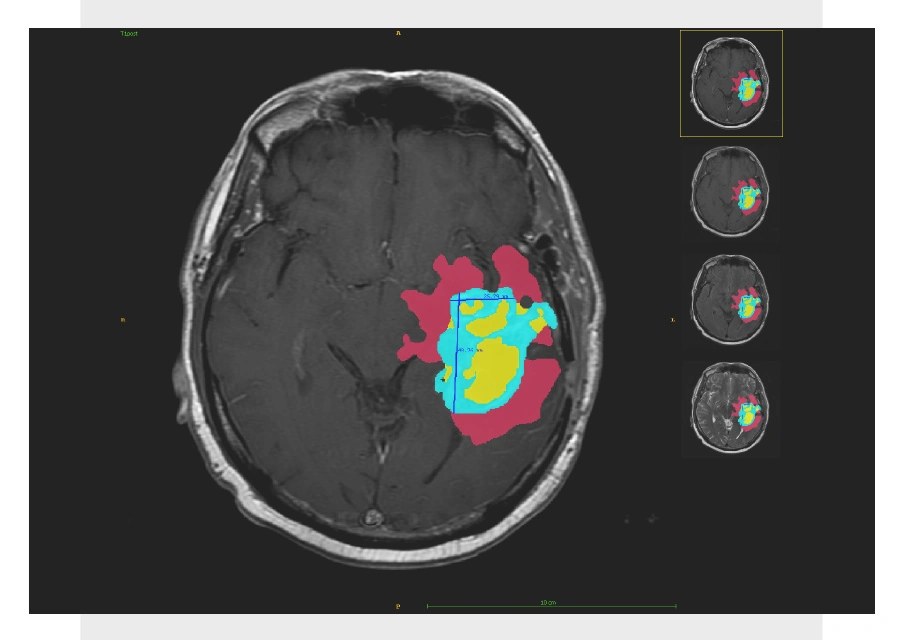
Automated segmentation of brain tumors with subregions
The above example displays how our algorithms have been used to assess the segmentation course of treatment as well as the progression of brain tumors.
Automatic brain tumor segmentation performed by our models – segmentation of 3 tumor subregions (edema, enhancing tumor and necrosis) and with additional volume and RANO measurements.
Depending on your unique needs, we can create specialized automated and semi-automatic algorithms to analyze both the brain and brain lesions.
Our models can be used to automatically monitor tumors in longitudinal studies and extract valuable information about the response.
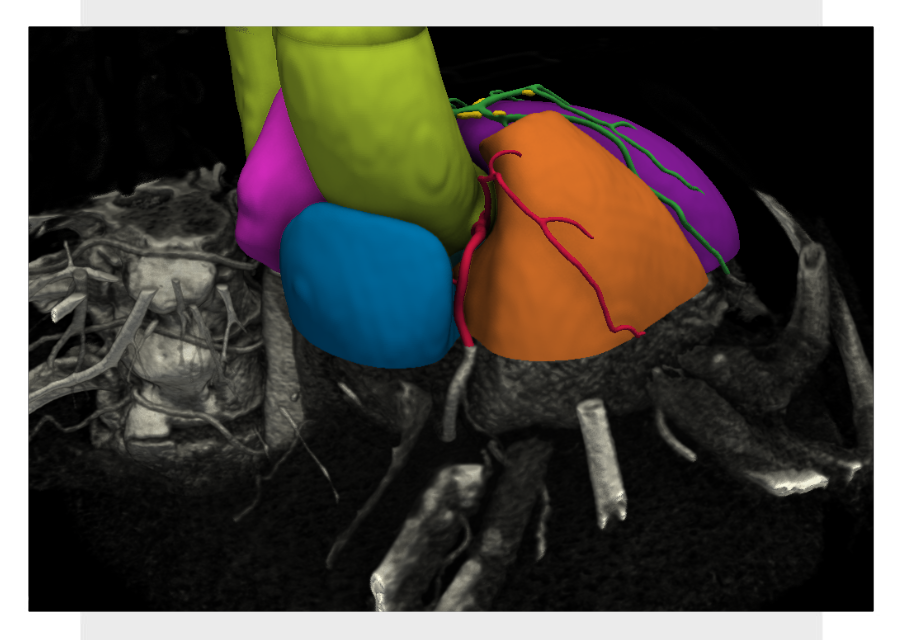
Cardiovascular imaging software and algorithms development
Within measurement in medical imaging, we proposed a comprehensive procedure to automate aortic stenosis and valve calcium scoring in cardiovascular imaging. In order to eliminate false positives (calcifications outside the valve) in cardiac CT scans, we implemented a tailored PSO algorithm.
We’ve created an AI-based method that uses deep learning to extract critical features for both the segmentation and quantification of coronary calcifications.
Our AI method successfully segmented the thoracic aorta on contrast-enhanced cardiac CT images. What’s more, an average Sørensen-Dice is over 0.98. We can say additionally, that with an open valve, the model performs well and yields accurate results over a range of image quality.
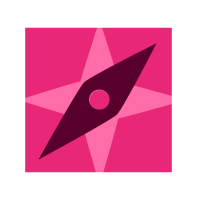
Benefit from AI measurement in medical imaging
Artificial intelligence in medical imaging has a significant impact on making precise measurements, which are vital for diagnosing diseases, monitoring changes over time, and also planning treatment.
- AI models extract quantitative data from medical images, ensuring a higher level of precision.
- AI plays a crucial role in both lesion detection and segmentation within medical imaging.
- AI-based models help track and measure lesion size, shape, and density over time, providing at the same time valuable quantitative data for monitoring disease progression or treatment response. This enables the development of personalized treatment plans.
- Manual image analysis is prone to variability in interpretation among different clinicians. However, AI-based measurement tools eliminate this variability by consistently applying standardized protocols across all scans, leading to more reliable data to support diagnosis and treatment based on repeatable measurements.
In sum, by embracing AI-based measurement in medical imaging tools, the medical professionals can fully leverage the potential of medical imaging to convert raw data into actionable insights.
Pixel power and data volume
500 exabytes of data are produced annually by the healthcare sector, with medical imaging accounting for the majority of this data. Moreover, a billion pixels can be produced from a single MRI scan. So, AI-based models process this massive volume of data to find anomalies. Under those circumstances, medical imaging deep learning models might have millions or even billions of parameters, which enables them to recognize complex patterns in medical images.
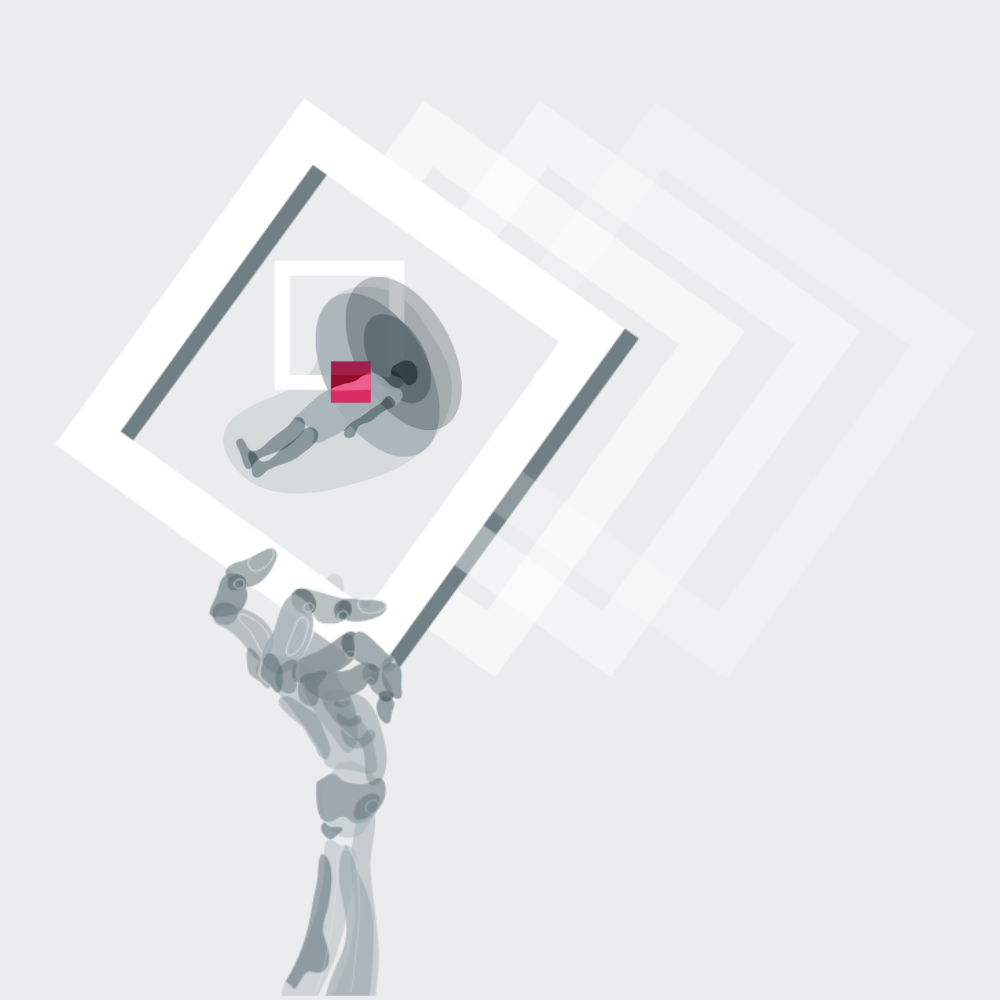